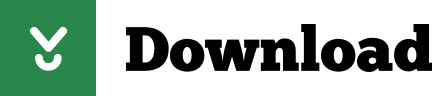
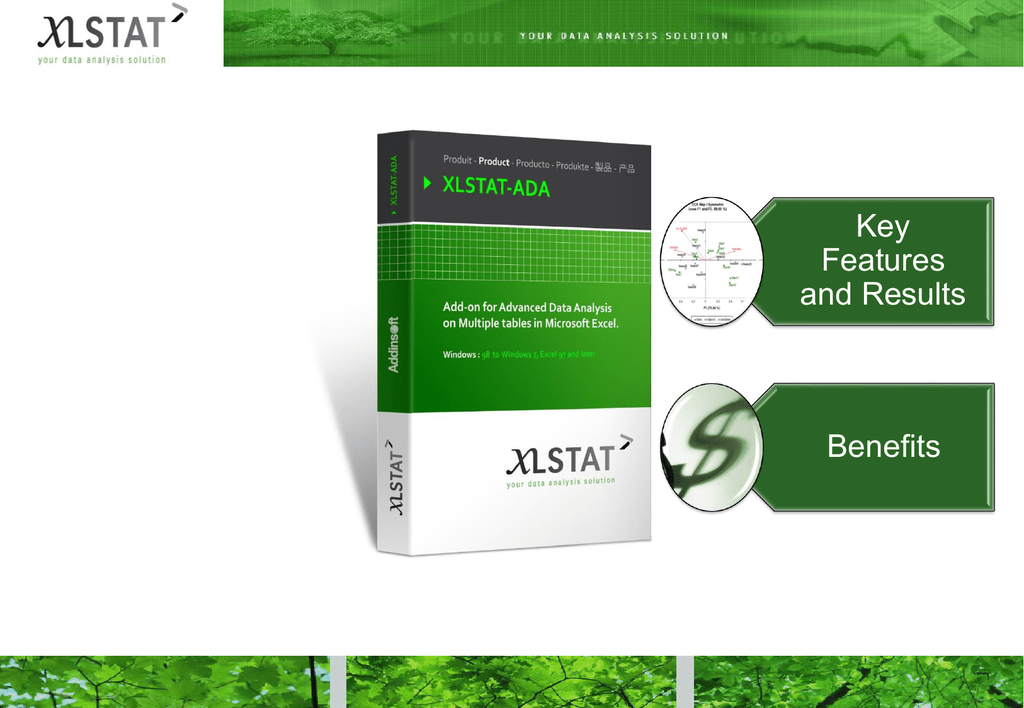
If a PCoA axis has a negative eigenvalue associated with it, imaginary numbers are generated during the analysis and prevent Euclidean representation.
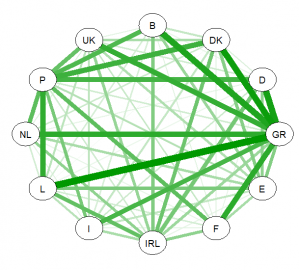
This may be used as a measure of the original variables' contribution to a given PCoA axis. However, object scores along the PCoA axes may be correlated with object scores along each original variable's axis, assuming the these are either quantitative or dummy variables (Legendre & Legendre, 1998). This is because PCoA takes a (dis)similarity matrix derived from the original data as input and not the original variables themselves. While PCoA is suited to handling a wide range of data, information concerning the original variables cannot be recovered.
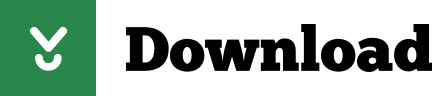